Understanding Theoretical Probability: A Comprehensive Exploration
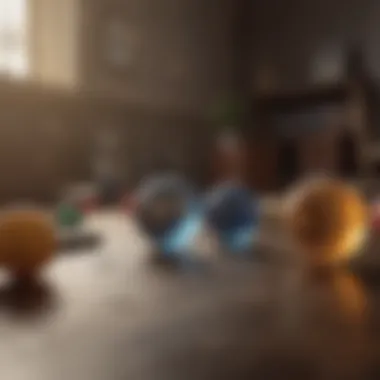
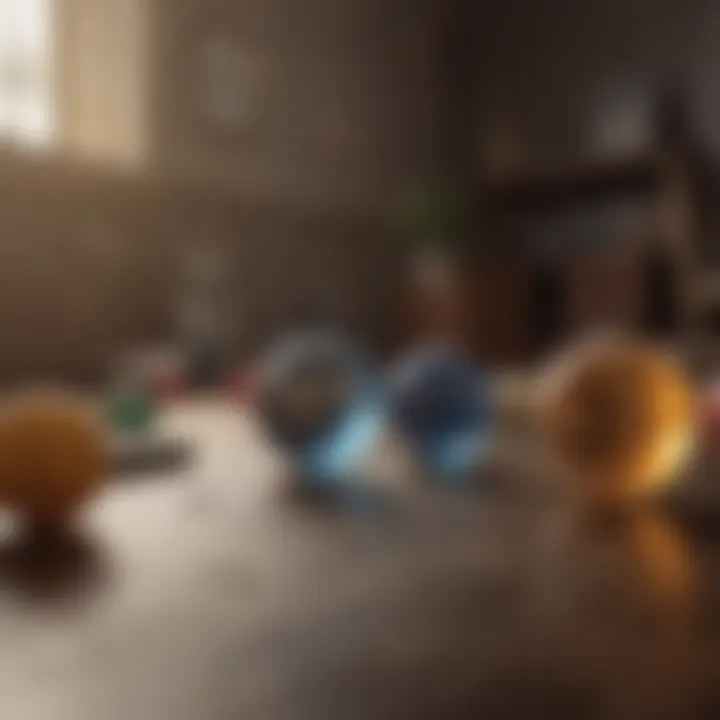
Intro
The concept of theoretical probability is essential in understanding how likelihood and risk shape financial decisions. This field, while seemingly complex, is rooted in straightforward principles that can be applied across various financial landscapes. Investors and financial enthusiasts often need a clear grasp of these principles to make informed choices, especially when assessing potential risks associated with investments. This article aims to guide readers through the intricacies of theoretical probability, contrasting it with empirical probability and illustrating how its principles can enhance risk assessment.
Key Terms and Definitions
Before diving deeper, it is crucial to grasp some key terms associated with theoretical probability, particularly within the investment realm. These definitions lay the groundwork for understanding how theoretical probability functions in practical scenarios.
Investment Concepts
- Theoretical Probability: Refers to the calculated likelihood of an event occurring based on the possible outcomes. In finance, it provides a framework for evaluating the expected success of an investment.
- Empirical Probability: This refers to the probability determined through actual experiments or observations, contrasting with the theoretical framework.
- Risk Assessment: The process of identifying and analyzing potential risks that could negatively impact an investment decision. Understanding theoretical probability limits uncertainty in this process.
Financial Instruments
Financial instruments are the tools investors use to engage in markets. Understanding their probabilities can help in managing risks effectively:
- Stocks: Shares of ownership in a company, where theoretical probability can indicate the likelihood of price changes.
- Bonds: Debt investments where the issuer owes a debt to the bondholder. Probability plays a role in assessing default risks.
- Derivatives: Financial contracts whose value depends on the performance of an underlying asset, with probabilities helping to estimate future pricing movements.
Expert Advice
Incorporating theoretical probability in financial decisions is not solely for academics; it has practical applications in investment strategies. Below are key considerations for both long-term and short-term investors.
Long-term vs. Short-term Investing
- Long-term Investing: This strategy benefits from theoretical probability as it can highlight trends and fluctuations over extended periods. Investors must consider market volatility and the associated probabilities of reaching financial goals.
- Short-term Investing: This approach involves quicker decision-making, where theoretical probability assists in estimating immediate market movements. Short-term investors can apply it to evaluate potential gains or losses based on historical behavior.
Risk Management Strategies
Implementing effective risk management requires a strong understanding of theoretical probability. Here are some strategies:
- Diversification: Spreading investments across various assets lowers the probability of significant losses.
- Hedging: Utilizing financial derivatives to offset potential losses in investments, informed by probability analysis.
- Analytical Tools: Tools like Monte Carlo simulations use theoretical probability to predict the probability of different outcomes, aiding investors in making sound decisions.
"Theoretical probability not only serves as a mathematical tool but also enriches investors' strategic thinking, allowing them to navigate uncertain waters with more confidence."
By understanding these foundational concepts, investors can apply theoretical probability more effectively, enhancing their ability to analyze and assess investment opportunities.
Prolusion to Theoretical Probability
The realm of theoretical probability serves as a fundamental cornerstone for understanding uncertainty in various fields, especially finance. As decision-making increasingly relies on probability assessments, having a firm grasp of theoretical principles becomes essential for conducting informed analyses. Decoding the nature of theoretical probability enables investors and financial analysts to model risk, make predictions, and interpret financial data with clarity. The nuanced understanding of this topic supports the entire framework for assessing potential outcomes and enhances the ability to navigate complex financial landscapes.
Definition of Probability
Probability is defined as a mathematical representation of the likelihood of an event occurring. It quantifies uncertainty and is expressed as a number between 0 and 1, where 0 indicates impossibility and 1 indicates certainty. In practical terms, probability allows us to make educated guesses about future events based on known information.
For example, when evaluating potential stock price movements, an investor may refer to historical data to determine the likelihood of a price increase or decrease. In such cases, the framework of theoretical probability provides essential methods to ascertain these likelihoods systematically.
Importance in Financial Decision-Making
Theoretical probability plays a crucial role in financial decision-making. Accurate probability assessments lead to better investment choices, risk management strategies, and overall financial literacy. By utilizing theoretical probability, investors can transform uncertain data into actionable insights.
The benefits of incorporating theoretical probability into financial decisions include:
- Risk Assessment: Identifying and quantifying risks associated with investments helps to make more rational decisions.
- Predictive Analytics: Utilizing probability calculations assists in forecasting market trends and making informed investment decisions.
- Portfolio Management: Probability aids in optimizing asset allocation by analyzing the expected returns and risks inherent in various financial instruments.
"Understanding probability is essential for making informed decisions in finance."
Thus, the principles of theoretical probability not only aid in risk assessment but also enrich the analytical framework essential for successful financial endeavors.
Mathematical Foundations of Theoretical Probability
Understanding the mathematical foundations of theoretical probability is crucial for comprehending how probabilities are calculated and applied. This section breaks down core principles, aiming to provide clarity on fundamental ideas that inform decision-making in finance and beyond.
Basic Probability Concepts
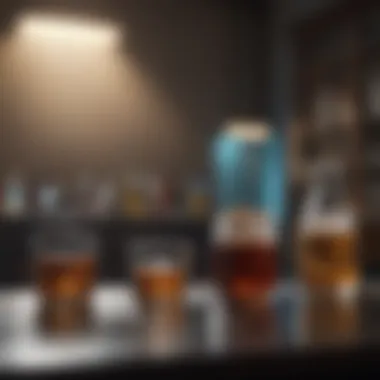
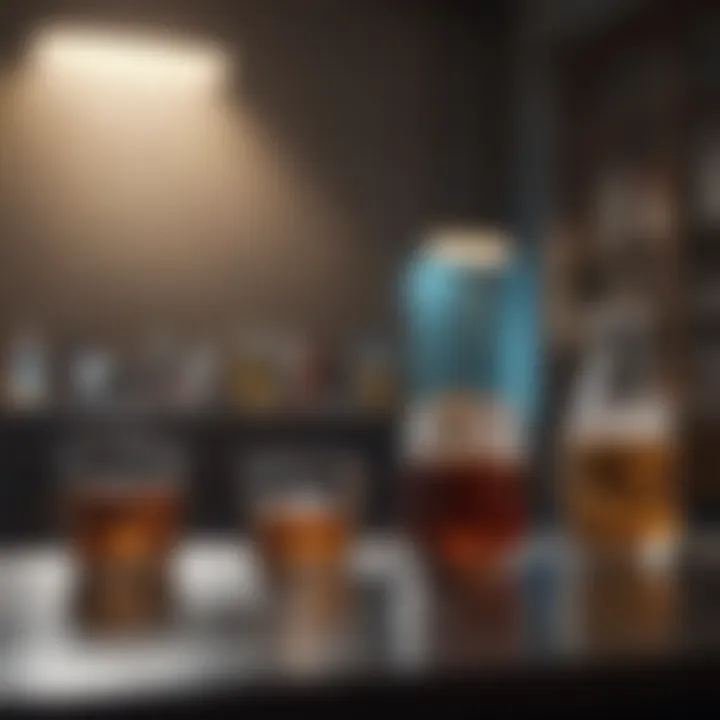
Probability is a way of quantifying uncertainty. It ranges from 0 to 1, where 0 indicates impossibility and 1 indicates certainty. The key concepts include:
- Experiment: An action or process that leads to one or more outcomes.
- Outcome: A specific result of an experiment. For example, rolling a die can result in any number from 1 to 6.
- Event: A set of outcomes. An event can consist of one or many outcomes.
These ideas form the backbone of probability theory, providing a language for discussing randomness.
Probability Formulas and Theorems
Understanding formulas and theorems is essential for applying probability effectively. Two fundamental concepts are the Addition Theorem and the Multiplication Theorem, each serving specific contexts in probability calculations.
Addition Theorem
The Addition Theorem deals with finding the probability of the occurrence of at least one of several events. It helps in computing probabilities in scenarios involving multiple outcomes. A key aspect of the Addition Theorem is its capacity to simplify calculations involving mutually exclusive events. This theorem is beneficial because of its straightforward application in various situations.
- Key Characteristic: It allows calculation of combined probabilities efficiently.
- Unique Feature: It is particularly useful when dealing with non-overlapping events.
- Advantages: Provides a clear method to calculate union probabilities.
- Disadvantages: Can complicate calculations in cases where events are not mutually exclusive.
Multiplication Theorem
In contrast, the Multiplication Theorem is focused on independent events. It states that the probability of the occurrence of two independent events happening simultaneously is the product of their individual probabilities. This theorem is popular among investors as it simplifies the computation of multiple independent events occurring.
- Key Characteristic: Allows easy calculations of joint probabilities.
- Unique Feature: It emphasizes independence between events, allowing simplification in calculations.
- Advantages: Facilitates analysis involving sequences of independent events, such as multiple trades in investment strategies.
- Disadvantages: Does not apply if events are dependent, which is common in financial scenarios.
Understanding these foundational mathematical concepts equips investors and financial enthusiasts with necessary tools to engage with theoretical probability effectively. By mastering these principles, individuals can better assess risks and make informed decisions in their financial endeavors.
Theoretical Probability Calculations
The field of theoretical probability calculations is crucial for understanding how probabilities work in various contexts. This segment emphasizes quantifying likelihoods of various outcomes based on initial assumptions, which is essential for making informed decisions, especially in finance.
Calculating probabilities involves determining the chances of certain events occurring based on known factors. This process aids in risk assessment during investments and can significantly impact financial decision-making. Accurate probability calculations can influence strategies and portfolios in investments, leading to better financial outcomes.
Understanding theoretical probabilities involves grasping several foundational concepts, among which are sample spaces, types of events, and how to compute probabilities effectively.
Sample Space and Outcomes
In probability theory, the sample space refers to the set of all possible outcomes of an experiment. For instance, if you flip a coin, there are two possible outcomes: heads or tails. Understanding this concept is fundamental, as it forms the basis for calculating probabilities.
When analyzing an investment scenario, determining the sample space allows financial analysts to foresee potential market behavior under varied conditions, helping to devise strategic responses to different market stimuli.
Event Types
Event types categorize how outcomes interplay within the sample space. This section discusses three main types of events and how they relate to the overall probability framework.
Independent Events
Independent events are occurrences where the outcome of one event does not affect another. A classic example is rolling a die and flipping a coin. The result of the die does not influence whether the coin lands heads or tails.
This characteristic is beneficial in establishing straightforward calculations. The unique feature of independent events lies in their easily calculable nature, which allows investors to evaluate risks without the added complexity of dependencies. However, one must be cautious; too much reliance on independence may lead to overlooking potential correlations in real-world scenarios.
Dependent Events
Dependent events involve situations where one event influences the likelihood of another. For example, drawing cards from a deck without replacement constitutes a dependent event. If you draw one card, the chances of drawing another specific card changes based on the first card drawn.
The understanding of dependent events is useful for detailed risk analysis where sequences of outcomes matter. A unique feature of dependent events is their dynamic nature, which requires ongoing assessment. The potential disadvantage here is the need for more comprehensive calculations, as each outcome influences the next.
Mutually Exclusive Events
Mutually exclusive events cannot occur simultaneously. For instance, in a scenario where one must choose between investment in stocks or bonds, selecting one eliminates the option of the other.
The hallmark of mutually exclusive events is their clear separation, presenting a straightforward decision-making scenario. This makes them popular choices for basic probability problems. However, their limitation lies in their binary nature, potentially oversimplifying more complex investment situations.
Calculating Probabilities
Calculating probabilities involves using the foundational understanding of the sample space and event types. The most basic formula is:
P(A) = Number of favorable outcomes / Total number of outcomes
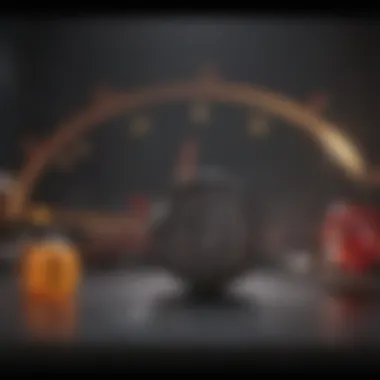
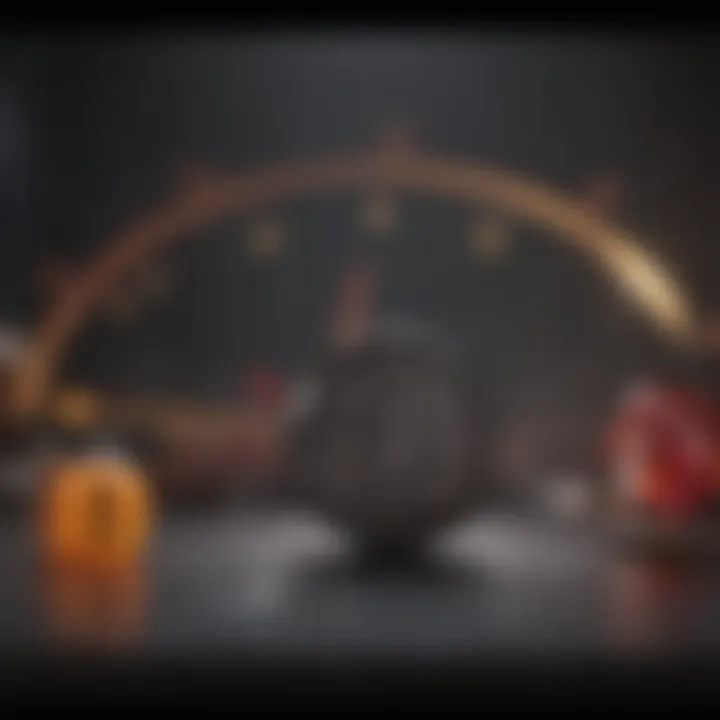
Where P(A) represents the probability of event A occurring. This formula lays the groundwork for more advanced calculations incorporating various theorems such as the Addition and Multiplication Theorems.
In practical scenarios, using these calculations can ultimately minimize risks and maximize returns in investment portfolio strategies, leading to more informed and strategic financial decisions.
Theoretical vs. Empirical Probability
The distinction between theoretical and empirical probability is critical for understanding how probability functions within different contexts, especially in finance. Theoretical probability defines what should happen in an ideal scenario, while empirical probability is based on actual outcomes observed in real-world experiences. This difference helps investors make informed decisions, as each type has its unique strengths and applications.
Defining Empirical Probability
Empirical probability, also known as experimental probability, refers to the likelihood of an event based on observed data rather than theoretical predictions. It is calculated by taking the ratio of the number of times an event occurs to the total number of trials conducted. For example, if a die is rolled 100 times and the number 3 appears 30 times, the empirical probability of rolling a 3 is 30/100 or 0.3. This data-driven approach can be particularly useful in fields where past behavior can predict future outcomes, such as in stock market trends.
Key Differences
Understanding the key differences between theoretical and empirical probability can influence decision-making significantly:
- Basis of Calculation:
- Nature of Data:
- Applications:
- Theoretical probability relies on predefined models and assumptions about the likelihood of events.
- Empirical probability is grounded in real data from actual experiments or observations.
- Theoretical probability often assumes ideal conditions where every outcome has consistent and quantifiable possibilities.
- Empirical probability reflects variability and unpredictability in real life, accommodating anomalies and irregular patterns.
- Theoretical probability is prominently used in mathematical modeling, offering a clean and logical framework for calculating risks.
- Empirical probability is beneficial for risk assessment, allowing investors to consider actual market behavior over time.
Theoretical probability provides a foundation; empirical probability captures reality.
When to Use Each Type
Both theoretical and empirical probabilities have their place in analysis and decision-making:
- Use Theoretical Probability When:
- Use Empirical Probability When:
- You need quick estimates or want to understand potential risks in an idealized setting.
- The events are controlled or predictable, such as in games of chance.
- A mathematical model or formula can be effectively applied.
- You possess historical data that can provide insights into current trends or behaviors.
- The situation involves complex systems with many variables, typical in financial markets.
- You aim to capture the nuances and variations of real-world events.
The choice between these two types of probability ultimately depends on the specific needs of the analysis and the context of the decisions being made. By understanding when to leverage theoretical probability versus empirical probability, investors can enhance their strategies and manage risks more effectively.
Applications of Theoretical Probability
The applications of theoretical probability span various fields, with finance standing out prominently. Understanding how theoretical probability works in practical scenarios can yield significant advantages for investors and financial analysts. By encapsulating the uncertainty associated with different outcomes, theoretical probability allows for more informed decision-making. It offers a structured approach to evaluating risks and potential rewards, making it indispensable in the finance sector.
In Investment Strategies
The use of theoretical probability in investment strategies is crucial. Investors rely on probability to assess potential returns, gauge risks, and create diversified portfolios. For instance, when analyzing stocks, theoretical models may predict the likelihood of a stock's price movement based on historical data and market conditions. This information helps investors to select stocks that align with their risk tolerance and investment goals.
A few ways that theoretical probability informs investment strategies include:
- Risk Assessment: By calculating the probability of various outcomes for an investment, investors can better understand the risks involved.
- Portfolio Diversification: Theoretical probability helps in constructing portfolios that aim to maximize returns while minimizing risks through the distribution of assets.
- Historical Analysis: Investors use past performance data to create theoretical models that predict future trends, guiding their investment choices.
In Risk Assessment
Risk assessment is another vital area where theoretical probability shines. It equips analysts with tools to quantify the risk associated with financial decisions. This is particularly important in situations where uncertainty looms large. Investors can utilize probability to evaluate the potential for loss and determine how to mitigate it.
Some key aspects of using theoretical probability in risk assessment involve:
- Value at Risk (VaR): This statistical method estimates the maximum potential loss over a specified time frame, under normal market conditions. It relies heavily on theoretical probability calculations.
- Stress Testing: Analysts use theoretical probability to simulate various adverse market scenarios. This helps institutions understand how their portfolios would perform under stress.
- Scenario Analysis: Probability allows analysts to create different scenarios based on varying assumptions, helping organizations anticipate potential issues and adjust strategies accordingly.
In Predictive Modeling
Predictive modeling is essential in forecasting future events based on historical data. Theoretical probability serves as a foundation for these models, blending statistical evidence with the likelihood of future occurrences. Financial institutions frequently employ this approach to improve their forecasting accuracy.
The following components highlight the role of theoretical probability in predictive modeling:
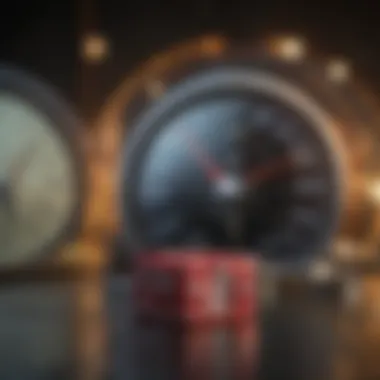
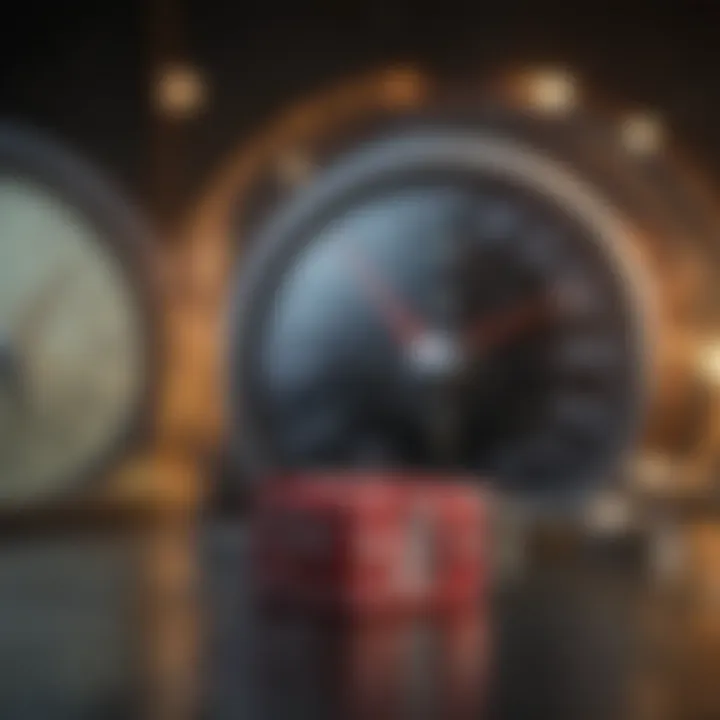
- Statistical Techniques: Techniques such as regression analysis rely on the principles of probability to draw conclusions about correlations and trends.
- Machine Learning Models: Many machine learning algorithms incorporate theoretical probability to predict outcomes more accurately based on prior data.
- Market Behavior Analysis: By analyzing patterns and associating them with probabilities, investors can make educated guesses regarding future market trends.
Understanding how theoretical probability applies in finance equips investors with insights to navigate complex markets effectively.
The Role of Theoretical Probability in Financial Markets
The concept of theoretical probability plays a crucial role in financial markets. It enables investors to make informed decisions based on calculated risks. Understanding the principles of this type of probability allows for better analysis of potential market movements and investment outcomes. Theoretical probability helps in quantifying uncertainty, which is inherent in all financial transactions. Here, we will explore its significance through market predictions and option pricing models.
Market Predictions
Market predictions are guided by theoretical probability. Investors analyze historical data and market trends to forecast future movements. For example, theoretical probability can estimate the likelihood of a stock price increasing or decreasing based on various factors. This allows traders to develop strategies that align with their risk tolerance. With sound predictions, investors can allocate resources more efficiently, reducing the probability of incurring unexpected losses.
Key components influencing market predictions include:
- Historical Data: Past performance can offer insights into probable future worth.
- Market Trends: Current trends often correlate with theoretical predictions.
- Economic Indicators: Factors like GDP growth or employment rates can impact probabilities.
By assessing these elements through the lens of theoretical probability, investors can harness the insights necessary for effective decision-making in volatile markets.
Option Pricing Models
Option pricing models incorporate theoretical probability to evaluate the value of financial derivatives. This section will cover two popular models: the Black-Scholes model and the Binomial model.
Black-Scholes Model
The Black-Scholes model is fundamental in the financial world, allowing for the determination of the theoretical price of options. It leverages assumptions about market behavior to create a framework for evaluating option prices. One key characteristic of this model is its ability to predict the option prices based on current stock price, exercise price, time until expiration, risk-free rate, and volatility of the underlying asset.
A distinct feature of the Black-Scholes model is its reliance on continuous-time finance and the normal distribution of asset prices. This provides significant advantages in terms of simplicity and efficiency in pricing options. However, it also has disadvantages, such as assuming constant volatility which may not reflect real market conditions.
Binomial Model
The Binomial model presents another approach to option pricing. It divides the price path of the underlying asset into discrete time intervals, leading to a tree-like structure of price changes. This model is particularly useful when dealing with American options, which can be exercised at any point before expiration. The key characteristic of the Binomial model is its flexibility in accounting for varying assumptions about volatility and dividends.
A unique feature of the Binomial model is its ability to handle multiple paths an asset's price can take. While it can be computationally intensive, this model results in a more nuanced understanding of options pricing compared to Black-Scholes. The trade-off may come in complexity, but for some investors, this precision offers a clear advantage.
The choice of option pricing model can have substantial implications for investment strategy and risk assessment, thus highlighting the overall relevance of theoretical probability in this context.
Critiques and Limitations of Theoretical Probability
The study of theoretical probability holds vast significance in mathematics and various practical applications. However, it is essential to recognize its critiques and limitations. Understanding these aspects not only informs the analysis of probability itself but also shapes its effective use in real-world scenarios. Investors and financial analysts who grasp these limitations can make more informed choices embedded in a probabilistic framework.
Assumptions in Probability Theory
Theoretical probability relies on several key assumptions. These assumptions create a model that aims to simplify complex realities. For instance, one common assumption is that outcomes are statistically independent. In financial markets, this is not always the case. Various factors influence market movements, affecting the independence of outcomes. Other assumptions include homogeneity of conditions and constant probabilities, which may not hold true in dynamic environments. These simplifications can cause theoretical models to diverge from actual results, leading to overconfidence in predictions.
Incorporating realistic assumptions may help improve models. However, this often increases complexity and may not align with simpler theoretical frameworks. The balance between simplicity and realism is a critical challenge in probability theory.
Real-World Implications
The limitations of theoretical probability have important implications for decision-making, especially in finance. Investors may rely heavily on theoretical models when formulating strategies or evaluating risks. When these models fail to account for market volatility or unforeseen events, the consequences can be severe. For example, risk assessments based on theoretical probability may underestimate potential losses in financial crises.
Furthermore, the reliance on historical data to inform theoretical models can mislead investors into overrating past trends as reliable indicators of future performance. Events like sudden market crashes or rapid technological changes often disrupt previous patterns, rendering traditional probability models inadequate. This disconnect can lead to misguided investments and, ultimately, financial loss.
"Understanding the limitations of theoretical models is essential for navigating real-world uncertainties."
To mitigate these risks, continuous evaluation and adjustment of models based on real-world feedback are crucial. Investors should remain adaptable and ensure their approach to probability integrates insights from empirical data and market behavior. It is through this nuanced understanding that one can better harness theoretical probability while acknowledging its inherent limitations.
Closure
In summarizing the exploration of theoretical probability, it is necessary to recognize its vast importance in various domains, especially in finance. This article has analyzed the theoretical aspects of probability, establishing its foundational concepts, contrasting it with empirical probability, and showcasing its significance in decision-making. Understanding these principles not only enhances one's analytical skills but also empowers investors and financial enthusiasts as they navigate complex markets.
Summary of Key Points
- Definition and Importance: Theoretical probability forms the basis for numerous financial models. It helps in predicting market trends and guiding investment decisions.
- Mathematical Foundations: The article elucidates core mathematical concepts that underpin theoretical probability. This includes essential formulas and theorems that facilitate accurate calculations.
- Applications: Real-world applications in investment strategy, risk assessment, and predictive modeling illustrate the practical utility of theoretical probability. Understanding these applications is crucial for effective financial planning.
- Critiques: The limitations and critiques of theoretical probability show that while it is a powerful tool, there are inherent assumptions that can affect its reliability in practice.
Future Directions in Probability Research
Future research in probability must address the complexities emerging from modern financial markets.
- Integration of Computational Techniques: Emerging methods, like machine learning, hold promise in enhancing probability models. These could lead to more accurate risk assessment and improved decision-making frameworks.
- Behavioral Finance: Exploring how human biases affect probabilistic reasoning is a critical area. This can provide insights into anomalies in market behavior that do not align with theoretical predictions.
- Enhanced Models: Continuing to refine and develop new models that encompass more variables will aid in applying theoretical principles to increasingly complex financial scenarios.
Continuous exploration in these areas will ensure theoretical probability remains relevant and valuable in an evolving financial landscape.